Bruce Rannala
Professor, Department of Evolution and Ecology @ UC Davis.
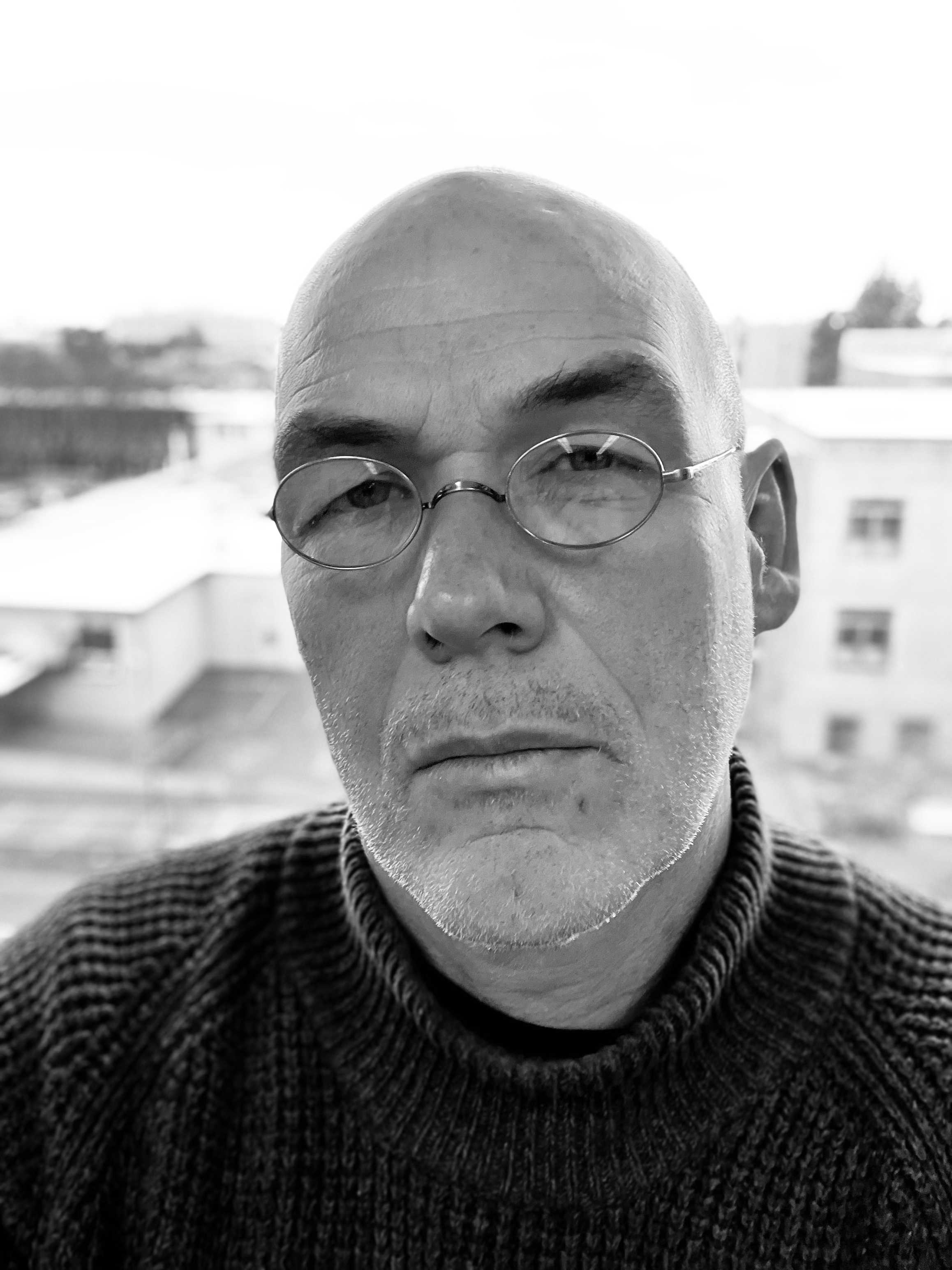
530-754-7729
Welcome to the home page of Bruce Rannala. I develop statistical methods, computer software, and sometimes new theory. I also teach a course on human genetic variation EVE 131 each Fall. My research focuses on statistical genetics, population genetics, and phylogenetics. However, our methods are often applied to real world problems such as pandemics (HIV, COVID19), cancer genetics, conservation biology and disease gene mapping.
Examples of Current Projects
- Bayesian inference of ages of latent lineages of HIV using sequence data (PhD student Anna Nagel)
- Bayesian inference of hybrid and backcross individuals using genomic sequences (Postdoc Sneha Chakraborty)
- New models aimed at early identification of SARS-CoV2 variants of concern using phylogenetic information (Postdoc Mike May)
news
Dec 18, 2023 |
Anna Nagel has received her PhD. ![]() ![]() |
---|---|
Oct 22, 2023 | Anna Nagel will present an exit seminar on her PhD work at the Center for Population Biology Seminar Series on November 14, 2023. |
Mar 14, 2023 | BayesAss (BA3) release 3.0.5.6 with new documentation and homebrew tap! |
Feb 16, 2023 |
Sneha Chakraborty has passed her PhD defense. ![]() ![]() |